Founder/CEO
- FMA
- The Fabricator
- FABTECH
- Canadian Metalworking
Pair your machine’s sensors with a computerized machine maintenance system
Condition-monitoring sensors reduce unplanned downtime, costs when connected to your maintenance system
- By Bryan Christiansen
- November 11, 2021
- Article
- Automation and Software
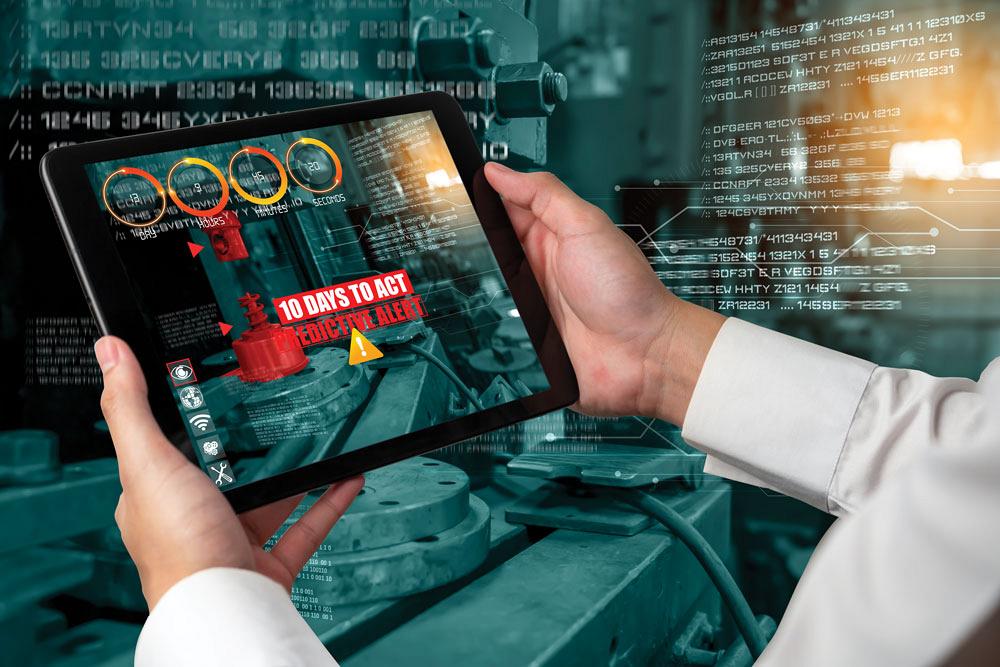
Increased productivity, reduced machine failures, greater worker safety, and reduced maintenance costs combine for efficient asset utilization. Getty
Industry 4.0 is a trending term. It describes the advent of the fourth revolution in manufacturing, integrating diverse new technologies to pursue efficiencies.
Small to medium-sized enterprises (SMEs) in the machining industry are slowly starting to rely on machine monitoring to maintain margins in a competitive manufacturing market that is suffering staff shortages and seeing a greater demand for customized products. Condition monitoring of machine tools coupled with predictive maintenance systems also can help deliver the required competitive edge.
The benefits include increased productivity, reduced machine tool failures, greater worker safety, and reduced maintenance costs. Let’s dive a little deeper into sensor technology for machine tools to understand how it works in practice.
Sensor Development
The Industrial Internet of Things (IIoT) has driven rapid advances in condition-monitoring sensor development. Modern sensors are packaged for harsh environments and use digitization, miniaturization, low power draw, and secure wireless connections. Advanced systems also allow sensor fusion, combining sensory data to reduce uncertainty in decision-making and intervention by maintenance personnel.
The eight most common outputs to measure are:
- Vibration
- Speed
- Current
- Temperature
- Pressure
- Fluid quality
- Fluid quantity
- Signal noise
Each of those categories includes multiple use cases.
For example, vibration sensors might measure the intensity, frequency, or amplitude of vibration in a rotating shaft. With all rotating machines having a distinct vibration signature, studying that signature and its increasing trend over time allows personnel to identify and rectify bearing wear, misalignment, rotor imbalance, and equipment looseness.
Once these problems are identified, maintenance technicians can avoid inevitable failures through interventions planned in downtime optimal to production. However, breakdown and maintenance improvements are not the only benefits.
Milling machines in particular benefit from vibration monitoring for operational optimization. All rotating spindles have a critical speed or natural resonant frequency, at which point the spindle suffers some distortion, rapidly increasing vibration. To maintain optimal cut quality and militate against accelerated wear of the spindle, operators must prevent sustained operation within the resonant frequency band.
Vibration sensors, coupled with speed sensor data, allow each spindle's critical speed to be calculated and then monitored. Operators may then manage the spindle speed to pass through the critical speed to more stable vibration levels for smoother operation.
Similarly, cut tool condition usually is detected by a deterioration of the surface finish on a component. Using vibration monitoring with AI creates machine learning that recognizes the point at which the vibration trend indicates a tool change is necessary before the visual impairment of the product.
Specific Sensor Applications
CNC machining centres:
- Acoustic emission sensors monitor cutting force for tool wear.
- Vibration sensors monitor vibration time and frequency changes for tool wear.
Milling machines:
- Vibration sensors monitor for toolholder misalignment.
- Semiconductor strain gauges monitor machining forces to minimize tool wear.
- Hall effect sensors measure motor current to monitor tool health.
Drilling machines:
- 3-axis force dynamometer and vibration sensors prevent drill failure in deep twist drilling.
- Temperature sensors detect imminent spindle bearing failure.
Tapping machines:
- Torque monitoring sensors detect tool wear and prevent thread damage.
Gear hobbing machines:
- Hall effect sensors detect spindle motor current draw reflecting increased tool wear.
- Temperature sensors detect cooling circuit failure through spindle heat.
Collecting Data
Operating in real time, the signals from the sensors pass through a wireless network to the cloud, where sophisticated condition-monitoring software captures and aggregates the data received from all installed sensors.
Creating time-series data, the software outputs continuous real-time operating and health parameters for equipment. Where exceedances from pre-established thresholds occur, alerts can be transmitted to maintenance personnel, informing them of the system deterioration.
Real-life Productivity Gains
Milling machine tool monitoring is a pertinent example of possible gains from using condition-monitoring sensors to improve product quality and production uptime. Cutting tools are critical components used in manufacturing to create metal parts during milling operations. Studies suggest1 cutting tools are typically used for only 50 to 80 per cent of their effective lifetimes, however. Causes of the shortened lifespan are excessive tool wear and breakage.
Tool breakages account for 7 to 20 per cent2 of total equipment downtime and 3 to 12 per cent3 of total processing cost. Therefore, breakages directly impact capital expenditure through increased tool spending and indirectly impact the bottom line through productivity losses.
Manufacturers employ many different condition-monitoring methods to manage tool use better and prevent breakage. As cutting force increases with tool wear, the milling machine motor current increases proportionally. With current sensors, real-time current use is passed to the cloud for analysis using artificial analysis and machine learning models.
The models can learn from in-use experience and detect a threshold beyond which tool breakage is imminent from the current trends. Such detection allows scheduled tool changeouts before the cut surface finish deteriorates and tool breakage occurs.
Benefits for the manufacturer are increased product quality, greater factory productivity, reduced capital expenditure, and a commensurate increase in earnings before interest, taxes, and amortization.
Condition Monitoring and CMMS
While condition monitoring can be operated as a stand-alone process, businesses can best leverage their gains by combining it with a computerized maintenance management system (CMMS). A CMMS collects historical operating and maintenance information to schedule planned maintenance or inspection tasks based on equipment hours, cycles, or calendar time.
With the evolution of technology, predictive maintenance techniques in a modern CMMS use machine learning to forecast future equipment conditions, scheduling maintenance interventions when they are most cost-effective for the manufacturer and before equipment condition deteriorates beyond a set level.
If a temperature sensor on a CNC machining centre passes data to condition-monitoring software indicating a rising trend in spindle bearing temperature, a notification is passed to the CMMS. The CMMS auto-generates a work order directing maintenance personnel to inspect and rectify, while potentially checking inventory for a replacement bearing. Depending on the AI analysis of severity, the work order priority can be scheduled for the next planned maintenance shutdown or on a priority basis for expedited attention. Urgent situations will generate instantaneous alerts to on-call maintenance personnel.
With manufacturing competitiveness shrinking margins, businesses are under increased pressure to automate their processes. As SMEs seek increased productivity, reduced machine failures, greater worker safety, and reduced maintenance costs, strategies for efficient asset utilization are critical.
Industry 4.0 technologies provide such a strategy, using condition-monitoring sensors linked to AI and machine learning algorithms. Such real-time monitoring linked to predictive maintenance techniques is already proving to be a game-changer for production facilities.
Notes
1. Konstantinos Salonitis, Athanasios Kolios, “Reliability assessment of cutting tool life based on surrogate approximation methods,” The International Journal of Advanced Manufacturing Technology (January 2014).
2. G. Vetrichelvan, S. Sundaram, S. Senthil Kumaran, P. Velmurugan, “An investigation of tool wear using acoustic emission and genetic algorithm,” Journal of Vibration and Control (February 2014).
3. C. Liu, G.F. Wang, Z.M. Li, “Incremental learning for online tool condition monitoring using Ellipsoid ARTMAP network model,” Applied Soft Computing (October 2015).
Bryan Christiansen is the founder/CEO of Limble CMMS, 3290 W. Mayflower Ave., Lehi, Utah 84043, 801-851-1218, www.limblecmms.com.
About the Author
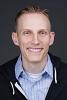
subscribe now
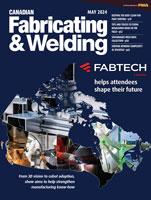
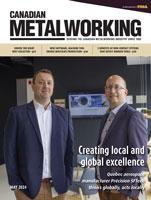
Keep up to date with the latest news, events, and technology for all things metal from our pair of monthly magazines written specifically for Canadian manufacturers!
Start Your Free Subscription- Industry Events
MME Saskatoon
- May 28, 2024
- Saskatoon, SK Canada
CME's Health & Safety Symposium for Manufacturers
- May 29, 2024
- Mississauga, ON Canada
DiPaolo Machine Tools Open House 2024
- June 4 - 5, 2024
- Mississauga, ON Canada
FABTECH Canada
- June 11 - 13, 2024
- Toronto, ON Canada
Zoller Open House & Technology Days 2024
- June 12 - 13, 2024
- Ann Arbor, MI